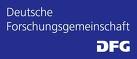
Hyperparameter Learning Across Problems
One of the major challenges of learning predictive models for complex tasks is the right hyperparameter and model selection strategy, as state-of-the-art approaches such as grid-search and random sampling require many runs of the learning algorithm, and therefore are usually conducted on large compute clusters rather than resource-restricted platforms such as robots, cars or mobile phones for instance. Therefore, autonomous hyperparameter learning strategies that are able to take into account observations of past hyperparameter performances on related problems have to be developed, enabling learning systems to learn in a fraction of the time it takes today.
In this project, we aim to design a hyperparameter recommendation model that is based on a priori characteristics of a new learning problem and few meta observations and is able to recommend further hyperparameter configurations to test, requiring only a fraction of runs of the learning algorithm, but still delivering comparable performance. This will be accomplished by learning latent features characterizing datasets and models by means of a factorization model in a way to directly correlate hyperparameter performance with these characteristics. Furthermore, active learning strategies will be employed to choose hyperparameter combinations to such an extent that the hyperparameter recommendation model demands even less runs of the learning algorithm.
The project will run for two years from 2014 - 2016.
Basic Information
Sponsor: German Research Foundation
Priority Programme: Autonomous Learning
HyLAP Homepage
Contact
Publications
- Martin Wistuba, Nicolas Schilling, Lars Schmidt-Thieme (2016):
Two-Stage Transfer Surrogate Model for Automatic Hyperparameter Optimization, in Proceedings of European Conference on Machine Learning and Principles and Practice of Knowledge Discovery in Databases (ECML'16), Riva del Garda, Italy. - Nicolas Schilling, Martin Wistuba, Lars Schmidt-Thieme (2016):
Scalable Hyperparameter Optimization with Products of Gaussian Process Experts, in Proceedings of European Conference on Machine Learning and Principles and Practice of Knowledge Discovery in Databases (ECML'16), Riva del Garda, Italy. - Martin Wistuba, Nicolas Schilling, Lars Schmidt-Thieme (2016):
Hyperparameter Optimization Machines, in Proceedings of IEEE International Conference on Data Science and Advanced Analytics (DSAA'16), Montreal, Canada. - Nicolas Schilling, Martin Wistuba, Lucas Drumond, Lars Schmidt-Thieme (2015):
Joint Model Choice and Hyperparameter Optimization with Factorized Multilayer Perceptrons, in 27th IEEE International Conference on Tools with Artificial Intelligence (ICTAI 2015), Vietri sul Mare, Italy. - Martin Wistuba, Nicolas Schilling, Lars Schmidt-Thieme (2015):
Sequential Model-free Hyperparameter Tuning, in Proceedings of IEEE International Conference on Data Mining (ICDM'15), Atlantic City, NJ, USA. Acceptance Rate: 18.2% (147 out of 807). - Martin Wistuba, Nicolas Schilling, Lars Schmidt-Thieme (2015):
Learning Hyperparameter Optimization Initializations, in Proceedings of IEEE International Conference on Data Science and Advanced Analytics (DSAA'15), Paris, France. - Nicolas Schilling, Martin Wistuba, Lucas Drumond, Lars Schmidt-Thieme (2015):
Hyperparameter Optimization with Factorized Multilayer Perceptrons, in Proceedings of European Conference on Machine Learning and Principles and Practice of Knowledge Discovery in Databases (ECML'15), Porto, Portugal. - Martin Wistuba, Nicolas Schilling, Lars Schmidt-Thieme (2015):
Hyperparameter Search Space Pruning - A New Component for Sequential Model-Based Hyperparameter Optimization, in Proceedings of European Conference on Machine Learning and Principles and Practice of Knowledge Discovery in Databases (ECML'15), Porto, Portugal. - Martin Wistuba, Nicolas Schilling, Lars Schmidt-Thieme (2015):
Learning Data Set Similarities for Hyperparameter Optimization Initializations, in Proceedings of the 2015 International Workshop on Meta-Learning and Algorithm Selection co-located with European Conference on Machine Learning and Principles and Practice of Knowledge Discovery in Databases 2015 (MetaSel@ECML'15), Porto, Portugal.